Data Governance Framework: Four Pillars for Success
Creating a data governance framework is a must-have for organizations that want to be truly data-driven. A data governance framework provides the essential structure that’s needed for the core elements of data management—data privacy and data security.
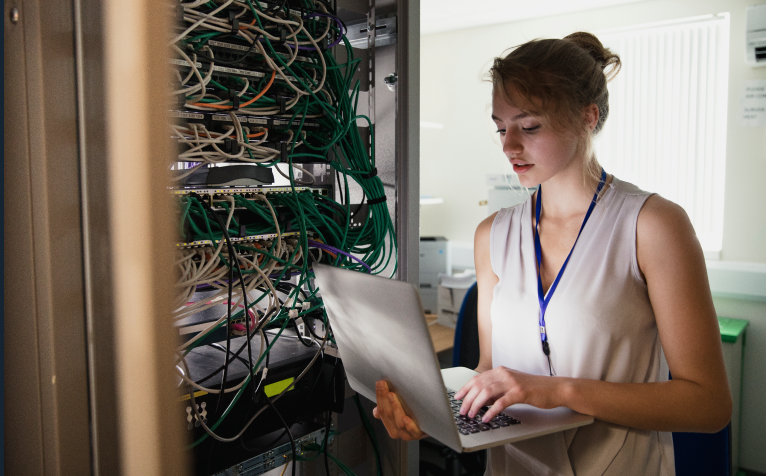
Despite the importance of prioritizing data governance, it is a difficult project for many organizations. A data governance framework eliminates the challenges by setting a clear path for building and maintaining a data governance program. It guides everything from identifying requirements and developing a plan to establishing a team and implementing the plan.
Done well, a data governance framework enables organizations to make the implementation of a data governance plan a smooth process that does not necessarily require a complete overhaul of existing data management systems. It helps organizations align and catalog existing data assets and establishes processes for ensuring that new data is organized and stored according to the data governance guidelines.
Let’s jump in and learn:
- Data Governance Defined
- What Is a Data Governance Framework?
- Benefits of a Data Governance Framework
- Goals of a Data Governance Framework
- Why an Organization Might Need a Data Governance Framework
- Four Pillars for Success in a Data Governance Framework
- Tips for Creating and Maintaining a Data Governance Framework
Data Governance Defined
Data governance is the practice of identifying, organizing, and managing data across an organization. It provides standards and procedures to keep data readily available and usable with data protection to ensure data privacy and data integrity. It is the decision-making function that overlays data management.
The collection of practices and processes that define the use of data are a key part of developing a data governance framework. By defining how data will be used and managed, a data governance framework helps organizations enforce rules that dictate data access and usage as well as provides direction on storage and retention. This is how data management assures optimal availability, usability, integrity, and security of the data across an organization.
A data governance framework directs how data will be managed and controlled. Among the areas commonly included in data governance frameworks are data policies, such as:
- Data availability
Accessible and ready for easy consumption.
Data qualities are retained, across different platforms, regardless of how it is stored, converted, transferred, and viewed.
- Data quality
Ensures data is correct, consistent, and cleaned.
- Data security
Safeguards are in place, including classification according to its sensitivity and storage policies and procedures that ensure data protection.
- Data usability
Clearly structured, documented, and tagged.
What Is a Data Governance Framework?
An actionable data governance framework includes rules, processes, and procedures to be followed on an ongoing basis. The primary objective is to enforce and facilitate data governance. In addition, a data governance framework details the components needed to implement and maintain a governance strategy.
A data governance framework should also include a statement of purpose, goals, and details for measuring success. It should spell out responsibilities and accountability for the various functions responsible for achieving the organization’s goals. All of that should be documented as part of the data governance framework and shared internally to ensure that everyone understands it and is committed to supporting it.
The structure of a data governance framework is meant to help organizations establish roles and responsibilities related to data, guide decision-making, and facilitate the use of data to maintain data quality and ensure data protection. Depending on the requirements, different types of data governance frameworks can be used. There are three types of frameworks:
1. Command and control—a small group of data stewards are assigned to take on data governance management responsibilities
2. Traditional—a larger number of employees are assigned as data stewards, with a few having additional responsibilities as critical data stewards
3. Non-invasive—individuals act as data stewards according to their existing work and relationship to the data (i.e., anyone who creates and modifies data becomes a data steward for that data)
A successful data governance framework has many components, including:
- Data stewardship
- Data quality
- Master and reference data management
- Data protection and security management
- Data privacy
- Data lifecycle management
Stakeholders in the Data Governance Framework
Including stakeholders in data governance framework development is crucial. These stakeholders should represent the different areas and functions within an organization. Stakeholders vary based on the structure of the organization and use cases, but generally fall into a few categories:
- Data governance
- Privacy and compliance
- Functional areas (e.g., IT, finance, operations) and lines of business
- Analytics and data science
Benefits of a Data Governance Framework
- Assures adherence to industry and regulatory compliance requirements
- Creates a golden record, master data management processes, and procedures for identifying and classifying data
- Enhances data quality
- Ensures data consistency across the organization
- Facilitates better decision-making and planning based on optimized data
- Implements controls that identify issues and keep data clean
- Provides procedures that guide how to regularly assess and consider adjustments to the framework to accommodate changes within the organization and to its data sources
- Strikes a balance between organizations’ operational need for flexibility and the controls that come with data governance and data management
Goals of a Data Governance Framework
It is important to establish the goals of a data governance framework before beginning to develop it. In detailing the goals, all constituents should be consulted to ensure that their needs will be met. Common goals of a data governance framework include the following.
Data Classification
A data governance framework can help organizations clearly understand the types of data they have as well as the value of it and its level of sensitivity (e.g., personally identifiable information or PII, protected health information or PHI). This makes it easier not just to find information, but also to employ sufficient data protection. In addition, by helping to classify information, data governance frameworks simplify the management of data lifecycles (i.e., data creation, data storage, data use, data archiving, and data destruction).
Data Protection
Data security plays a significant role in a data governance framework. Enhancing data protection is a key goal of most data governance framework projects. A data governance framework will cover a range of data protection and data privacy areas, including software and hardware protection, data access, storage, and authentication processes.
Data Quality
Data governance frameworks help organizations assess and maintain data quality.
Data Retention
A data governance framework includes processes for determining when good data becomes obsolete and should be destroyed.
Data Sharing
Data governance frameworks dictate the level of access and accountability that users have with content and ensure that the same data can be shared among multiple users and applications while maintaining data quality and data integrity.
Regulatory Compliance
One of the primary drivers behind data governance framework projects is to address compliance requirements, such as Sarbanes-Oxley Act, General Data Protection Regulation (GDPR), California Consumer Privacy Act (CCPA), and The Payment Card Industry Data Security Standard (PCI-DSS).
Why an Organization Might Need a Data Governance Framework
A data governance framework is crucial to getting the most out of data governance programs. It provides standards for organizations’ rules and establishes processes for storing, using, and collecting data. The data governance framework identifies data owners, creates catalogs, improves data availability, increases data literacy and access, and sets procedures to enforce data policies.
In addition, a data governance framework sets up data risk and privacy metrics, including key performance indicators (KPIs), to gain insight into the progress of adoption and workflows. It also provides details about how data is being used and who uses it, using technical metadata, data profiling and lineage, and collaboration tracking.
Putting a data governance framework in place gives organizations a detailed plan with processes to make data accessible, protect it, and ensure data quality. A data governance framework supports organizations in several other ways, including:
- Avoiding mishandling of data
- Controlling access to data, including revoking access when employees leave an organization, or a partner no longer requires the data
- Destroying obsolete data
- Identifying data governance issues
- Preventing issues that can lead to poor data quality, access, or controls
- Providing clear controls over data—across all users, applications, and storage scenarios
- Providing visibility into data accessibility and quality
Four Pillars for Success in a Data Governance Framework
There are four pillars to the data governance framework to enable organizations to get the most out of their data.
1. Identify distinct use cases
It is of the utmost importance to connect data governance to its impact on an organization by considering revenue, cost, and risk. Identifying use cases provides an opportunity to engage stakeholders and make them part of developing the data governance framework.
Getting stakeholders involved not only helps ensure that real, meaningful issues are being addressed, but also gets them invested in the project and committed to its success. Examples of use cases include:
- Revenue focused—grow the organization by using data to:
- Expand customer lifetime value
- Identify new customers and market opportunities
- Optimize marketing campaigns and promotions
- Speed time to market
- Target and specialize product and service offerings
- Cost focused—optimize operations by using data to:
- Eliminate duplicate data to reduce storage costs
- Increase sales and marketing efficiency
- Reduce operations and maintenance costs
- Risk focused—improve security and compliance by using data to:
- Adhere to regulatory compliance requirements
- Simplify auditing and reporting
- Enhance data protection by identifying all data sources and flagging sensitive data
The number of use cases for data governance is practically limitless. But the data governance framework development works best by starting with a solid use case that represents characteristics of most of the others. Then, take lessons learned from the initial use case to deploy the data governance framework across the organization.
2. Quantify value
It is important that the impact of the data governance implementation is measurable. The value of the data governance framework must be quantifiable in the individual use cases and for the organization as a whole.
Key performance indicators (KPIs) need to be established and reported out regularly to show the value delivered from the data governance framework. These will also help identify areas that require attention and improvement.
3. Improve data capabilities
The data governance framework should outline the capabilities that are needed to improve the value of data for users and address individual needs for data usage. This should include the technology and processes needed to empower users to make the most of available data to meet requirements that are set forth in the use cases. Capabilities that are commonly included are the ability to:
- Collaborate across the organization to share data assets
- Comply with internal and external regulatory compliance requirements
- Discover data assets with an intuitive and searchable catalog
- Provide secure data access based on the principle of least privilege, to enforce data protection
- Trust the accuracy of data
- Understand the data’s origin, classification, content, and use
4. Develop a scalable delivery model
After the initial use case is addressed, the data governance framework can be established as a scalable service. The effort should decrease with each new use case while delivering increased benefits. In this way, the data governance framework grows the return on investment as more use cases are added and, ultimately, it is applied across an organization.
Tips for Creating and Maintaining a Data Governance Framework
Mind the four pillars for success when dealing with a data governance framework, and remember that it is a strategic asset that should be developed, maintained, and protected with care on an ongoing basis. It should be treated as a practice that is embedded in all of an organization’s workflows.
Considerations and steps to take when creating a data governance framework include the following.
- Develop a data governance strategy
- Document existing processes, users, and workflows
- Identify where data is, how it is used, and what people or applications use it
- Gather data protection requirements
- Start small
- Select one area of the organization or a data issue to start with
- Begin with an area that has significant problems or imminent risks
- Measure results and document problems to optimize the data governance framework moving forward
- Select a data governance framework template that fits the organization’s data
- Use a template that aligns with the data governance strategy
- Ensure that the data governance framework can be integrated across the organization
- Communicate and collaborate
- Establish a communications strategy to inform users across the organization about the data governance framework and how it will work
- Engage data stakeholders in the data governance framework development
- Create a plan to maintain and update the data governance framework
- Track and measure performance
- Continue to make refinements to address issues and optimize processes
- Make refinements as requirements change
- Establish risk milestones
Perhaps most important is maintenance. A data governance framework should be a living process that grows and changes with organizations’ needs. Put processes in place to ensure that the data governance framework is regularly reviewed by all stakeholders and updated as needed.
Egnyte has experts ready to answer your questions. For more than a decade, Egnyte has helped more than 16,000 customers with millions of customers worldwide.
Last Updated: 9th March, 2022