Coding Qualitative Data Guide
What Is Coding in Qualitative Research?
Coding in qualitative research is the process of sorting and organizing non-quantified data. This involves separating a word or phrase and using a code to tag it. The code describes a portion of data and is used to separate the information into unique categories, allowing researchers to find and cluster related content quickly.
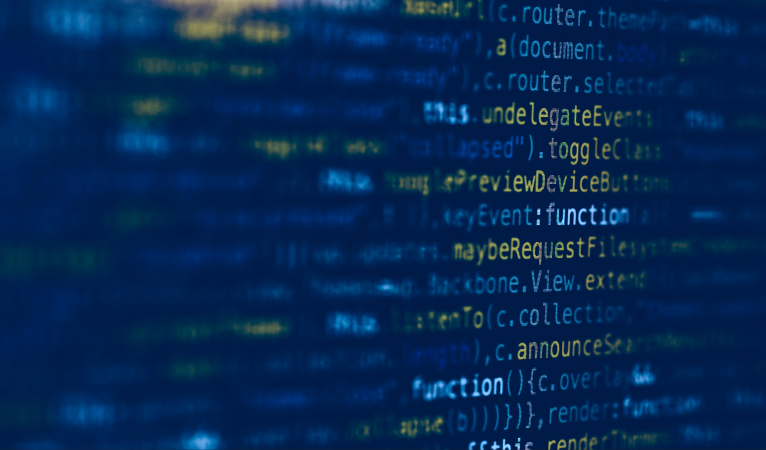
Categorized data can also be analyzed as a unique data set or linked with other coded data sets for analysis. The ultimate objective of coding qualitative data is to transform it into a format that can be used for research and reporting. In fact, coding is considered to be the first step of analysis.
Codes can be a word or a phrase that represents a recurring theme or idea in the data. The code name should be intuitive and reflect the essence of the participants’ responses or the researchers’ observations. These codes are created using two methods of coding qualitative data—automated coding and manual coding.
- Automated Coding of Qualitative Data
Automated coding uses qualitative data analysis software to review the data set, identify themes, and create codes. The software uses a combination of machine learning, artificial intelligence (AI), and natural language processing.
- Manual Coding of Qualitative Data
Manual coding requires researchers to read through the data and manually develop and assign codes and themes.
Questions to Consider when Coding Qualitative Data
- What does the response represent?
- What is the person saying?
- What is the response an example of?
- What is the source of the material (e.g., interview, questionnaire)?
- What patterns are emerging?
- What questions are trying to be answered with the research?
What Is Qualitative Research?
Qualitative research is the collection, analysis, and interpretation of non-numerical data (e.g., text, video, photographs, audio) to understand concepts, opinions, or experiences. It is used to gather subjective data, primarily through interviews and surveys, to explain “how” and “why” of a particular phenomenon or behavior.
Examples of Qualitative Research Questions
- How can teachers integrate nutrition into curriculums?
- How do children and adults interpret social issues?
- How does advertising shape body image in women?
- How is COVID experienced around the world?
- What factors influence employee retention?
Five Approaches in Qualitative Research
1. Ethnography
Studies people in their natural environment by immersing into the culture and observing participants in situ
2. Narrative
Examines how stories are told to understand how participants perceive and make sense of their experiences
3. Phenomenological
Uses a combination of methods, such as conducting interviews, reading documents, watching videos, or visiting places and events, to understand the meaning participants place on the topic being researched
4. Grounded theory
Uses interviews and existing documents to build a theory based on the data
5. Case study
Explores multiple data sources, including interviews, to explore, explain, or describe a person, place, event, or thing
Five Qualitative Research Methods
1. Observations
Recordings of what a researcher sees, hears, or encounters
2. Interviews
Asking people questions in one-on-one conversations
3. Focus groups
Asking questions and generating discussion among a group of people
4. Surveys
Gathering information through questionnaires with open-ended questions
5. Secondary research
Collecting data from existing texts, images, audio, video recordings, or social media
Types of Qualitative Data
The two types of qualitative data are nominal and ordinal.
1. Nominal data
Information that is observed, not measured. Nominal data includes items that are distinguished by a simple naming system.
There is no numeric value for nominal data. Examples of nominal data are country, gender, race, hair color, and profession.
2. Ordinal data
Information with natural, ordered categories and the distances between the categories are not known. An example of ordinal data is inputs related to satisfaction on the variable scale—satisfied, indifferent, dissatisfied.
Transcribing an Interview
Much of the qualitative data collected in studies is in the form of audio or video recordings gathered from a variety of sources, including interviews, focus groups, and talks during consultation. To make this information easier to review and organize, it is usually transcribed into written form.
While transcription is often thought to be a straightforward technical task, it actually involves judgments. Examples of this are:
- What level of detail to include, such as omitting non-verbal dimensions of interaction (e.g., facial expressions, gestures, paralinguistics such as loudness or tone of voice, body language, proxemics or personal space, eye gaze, haptics or touch, appearance)
- Data interpretation, such as distinguishing “I don’t, no” from “I don’t know”
- Data representation, such as representing the verbalization “hwarryuhh”as “How are you?”
In qualitative research, the representation of audible and visual data into written form is an interpretive process that involves making judgments. Therefore, it is considered to be the first step in the data analysis process. Because there are many different ways to transcribe the same data, the level of detail and different representations of data are driven by the aims and methodologies of the research project.
Transcribing is a time-intensive task. On average, transcribing qualitative research data can take at least three hours and up to ten hours per hour of audio or video. However, there are benefits to transcribing research data, including:
- Transcription provides a written record of research data
- More easily translated into other languages
- Offers a more objective look at the interview questions and responses
- Can be made available in more formats
- Offers more options for analyzing, storing, and sharing the information
- Requires less memory than storing audio or video
- Can be shared with fellow researchers, participants, or outside auditors more easily
- Transcription helps save time
- Easily search the text or timestamps for specific words or quotes
- Eliminate the need to play the audio or video recording over and over to find information
- Find the facts faster
- Transcription keeps research accurate
- Adhere to the standard protocol that qualitative research requires, including credibility, context, and transferability
- Do not have to rely on memory, handwriting, or typing speed
- Allows a more complete record of the interview questions and answers
Deductive and Inductive Approaches to Qualitative Coding
Before coding qualitative data, researchers need to determine which methods to use—deductive or inductive coding. Most studies use a combination of deductive and inductive methods.
Research objectives drive this decision about the primary method for coding qualitative data. Often researchers begin with a predefined list of codes, then inductively modify and add to the list as they parse the data.
Deductive Approach for Coding Qualitative Data
A deductive approach is a top-down way for coding qualitative data in which researchers formulate pre-set coding schemes. The coding schemes are usually derived from themes that come in related research reports or papers. The resulting set of codes are then applied to the qualitative data.
Inductive Approach for Coding Qualitative Data
An inductive approach is bottom-up. Researchers derive codes from the collected information using in vivo codes (i.e., words or phrases taken directly from the participants’ responses). The list of codes is created and modified as the qualitative data is processed.
Steps for Coding Qualitative Data
Coding qualitative data allows it to be organized in a way that can be more easily sorted for review and analysis. This involves assigning textual data (e.g., interview transcripts, notes, field observations) a code based on its category. The categories are derived from themes, ideas, and concepts related to the research as well as the participants’ own words or phrases.
Coding Qualitative Data at a Glance -Read through the text data -Divide the text into different groups of information -Label each group with codes -Reduce overlap and redundancy -Collapse code into themes |
Five Steps for Coding Qualitative Data
Step one: Review the data and create a storyline around the research question.
After reviewing qualitative data, consider the research question and develop a storyline around it. This storyline can be a couple of sentences or a short paragraph. Taking the time to develop a storyline before coding qualitative data has several benefits, including:
- Helps researchers decide what concepts and themes to highlight
- Guides how qualitative data should be organized and coded
- Provides a structure for the coding scheme
Step two: Develop a set of codes for qualitative data.
The number of codes will be driven by the amount of content and required granularity. When creating codes, keeping the number to the minimum required is recommended, because as the number of codes grows, so does the complexity of organizing the data. As codes are created, it is important to keep notes about their meaning and derivation as well as any connections to other codes.
Codes can be both pre-set (i.e., a priori) and open (i.e., emergent). Most researchers use a blend of both models.
- Pre-set codes
A list of pre-set codes generally ranges from as few as ten codes or up to fifty codes. These can be derived from a number of sources, including the storyline, list of research questions, and problem areas.
- Emergent codes
Codes that emerge from reading and analyzing the data are emergent codes. These include ideas, concepts, actions, relationships, and meanings.
Step three: Begin coding qualitative data.
Reread or listen to all the data and assign codes to general phrases or ideas that surface. After coding the data (i.e., words, phrases, sentences, paragraphs), the codes should be grouped into categories and developing themes.
Codes are sometimes grouped together according to similarity or by topic or general concept—aligned with the storyline. During this process, themes or patterns will emerge across the data set that supports the storyline and will become the narrative of the research.
Step four: Refine qualitative data coding.
After taking a break from the data, the codes and all of the information categorized under each should be carefully reviewed. During this process, codes will be added, deleted, revised, broken into sub-groups, or collapsed into one another.
Here, it is important to remember to make the codes fit the data and not vice versa. This step is often repeated several times.
Step five: Using memos for clarification and interpretation.
Once codes have been established, it is important to create a codebook or data dictionary that includes all notes related to each code.
Tools for Coding Qualitative Data
There are a number of tools for coding qualitative data ranging from manual and paper-based to digital options available as packaged software or as a service. Following are the three categories of tools for coding qualitative data. Regardless of the method that is used, in the end, the information gathered will be organized by theme and codes for use as part of the research.
Three Categories of Tools for Coding Qualitative Data
1. Manual Tools for Coding Qualitative Data
The manual method involves printouts of transcripts, scissors, and folders. This is a very “old-school” method, but it is effective for the right data sets and teams.
Basically, transcripts are printed, cut into snippets, and tagged with a code. The codes are organized into groups that are evaluated to determine which codes make the most sense in which group, based on the themes. Then, the snippets are put into folders according to themes.
2. Digital Tools for Coding Qualitative Data
A simple word processing and spreadsheet application (e.g., Microsoft Word and Excel, Google Docs and Sheets) can be used for coding qualitative data. The digital copies of the interview transcripts can be highlighted (i.e., using different colors to code the snippets from the transcripts) and comments used to tag the snippets with codes.
All of the codes should be summarized in the spreadsheet, then moved around and organized by theme. Then, additional pages should be added to the spreadsheet for each of the codes. The snippets should then be cut/pasted from the word processing document into the correct tab of the spreadsheet.
3. Purpose-Built Software and Services Tools for Coding Qualitative Data
Many companies offer a variety of software and services designed specifically to help with qualitative data coding, management, and analysis. These tools and services offer support for a number of tasks related to coding qualitative data, including transcription analysis and creating codes for source data (e.g., interviews, surveys, transcripts, focus groups, text/videos/audio files, images, and social media posts). The methodologies used for these qualitative data coding and analysis tools include open coding, an emergent coding technique drawn from grounded theory methodology template coding, and a priori coding technique drawn from template analysis.
Unleash Insights by Coding Qualitative Data
Coding is the key to extracting value from qualitative data. Without coding qualitative data, it is next to impossible to organize the unstructured content that makes up the bulk of qualitative data sets.
Developing a set of codes for qualitative data sets takes a significant amount of time and effort. However, every bit of time spent coding qualitative data yields a multitude of benefits—enriching research projects and providing rich resources for future use.
Egnyte has experts ready to answer your questions. For more than a decade, Egnyte has helped more than 16,000 customers with millions of customers worldwide.
Last Updated: 24th February, 2022