Data Centricity
Let’s jump in and learn:
- What Does Data Centric Mean?
- Characteristics of Organizations That are Data Centric
- Five Steps to Create a Data Centric Organization
- Four Data Centric Use Cases
- Benefits of Data Centricity
- What Is a Data Centric Model?
- Data Centric vs Data-Driven
- Why Is Data Centricity Important?
- Being Data Centric Delivers Value Across the Data Value Chain
What Does Data Centric Mean?
Data-centric (also referred to as data-centric architecture, data-centric infrastructure, or data-centricity) describes an architecture with data at its core. With data centric models, data is the main asset and remains persistent, while applications are deployed, updated, replaced, or retired. In this model, applications are transitory, but the data remains in place. Data centricity precedes the implementation of applications and stays in place for its entire lifecycle. As organizations move through a data maturity model, being data centric is the last stage.
Data science plays a core role in organizations that are data-centric. In these organizations, groups are dedicated to data-related programming and analysis. All decisions are driven by 360-degree views powered by data analytics. Data centricity gives the decision-makers a complete view of how the data is used across the business. Organizations with this commitment of resources to data are considered to be data-centric.
A few common roles in data-centric organizations are:
- Data engineers, usually in their own department, are responsible for storing data and ensuring the infrastructure is scalable. They are also responsible for extracting the relevant pieces of data and packaging up datasets for data scientists to analyze and mine for insights.
- Data science departments are focused only on the analysis of data and research. They do not get involved in software development or data engineering. The data scientists take the vast amounts of data that organizations gather and wrangle it using advanced analytics to provide actionable insights using machine learning and artificial intelligence. To be effective, data science should be considered one of the most important processes in the organization and must have a strong commitment from leadership in terms of enforcing protocols and providing the resources needed to support the program.
- Assigning the chief data officer role is also an integral part of data centricity. It demonstrates an organization’s seriousness about data and being data-centric. The chief data officer manages strict processes to support data science and delivers the results of advanced analytics to the various constituents and decision-makers across an organization.
For some organizations, the move to a data-centric model is precipitated by limitations encountered with an application-centric approach. Many organizations that originated as online-based began data centricity. Regardless of how or why an organization is data-centric, the objective is to put data in the top position.
Once deployed, data centricity should be baked into the culture as well as into the implementation of all systems. Instead of focusing on applications, data-centric organizations pay attention to finding ways to optimize the collection, processing, and management of data. Applications are built to fit in with data strategies and the prevailing rather than the other way around. IT teams in data-centric organizations design infrastructure around data requirements, rather than trying to make data fit the infrastructure. Data centricity allows organizations to eliminate data silos, simplify data management, and expedite application development because teams work within a framework that encompasses data and applications.
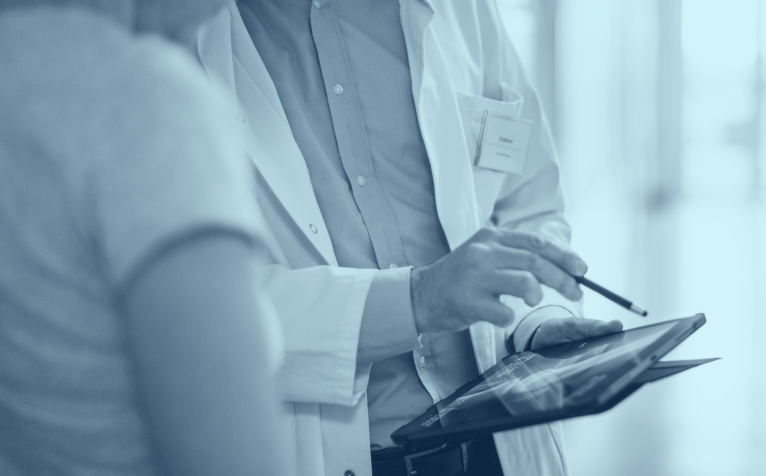
Characteristics of Organizations That are Data Centric
1. Aligned and committed to using data as the driving component of its strategy
2. Digital infrastructure is built around the optimal collection, processing, management, and distribution of data
3. Culture is focused on the value of data and has the requisite systems to support this
4. Programs are embedded into all aspects of the organization to support data, including data management and data governance
Five Common Components Required to be Data Centric
1. Strategic framework designed around data centricity
- Establishes a data framework that meets overall objectives and interprets data across all areas of an organization
- Allows data scientists and architects to provide the infrastructure that supports the data strategy
2. Data-centric workloads
- Provides agility and flexibility to ensure the utilization of all available data
- Supports the fluid movement, management, and utilization of unstructured datasets
3. Data and architecture aligned with data centricity
- Uses technology to support data-centric functions
- Measures how technologies can produce the necessary to generate meaningful data and measure its efficacy
4. Data governance for data centricity
- Determines how long data is stored, where it is stored, and the process for removing data once it reaches the end of its life cycle based on data lifecycle policies
- Ensures that it is secure, accessible, and high-quality
- Includes protocols for data breaches if they occur.
- Informs data access with a data org chart that documents that inform rules
- Meets regulatory, industry, and government standards, such as the European Union’s General Data Protection Regulation (GDPR), Health Insurance Portability and Accountability Act (HIPAA), and the Payment Card Industry Data Security Standard (PCI DSS)
- Provides security policies to determine how data is stored and protected
- Technology documentation that lists the technology platform used and how used
- Uses meta information to include descriptions within a site, app, or platform that help make information easier to find through search and tagging by providing a consistent format and semantics guidelines for updating meta information
5. Reporting and visualization
- Allows data scientists to create custom dashboards based on the organization’s role and level of access
- Determines which reporting tools and functionalities are available (e.g., how data is presented, if the data visualization is interactive)
- Presents data in a more understanding visual format to make it more digestible using charts, graphs, maps, and other illustrative formats
Five Steps to Create a Data Centric Organization
1. Communicate with stakeholders to explain the data strategy and how they can use best practices
2. Create a data culture harnessing data enthusiasts in the data analyst team and encouraging them to educate others about data culture
3. Practice sound data governance, developing a data governance framework to help with data profiling and data management
4. Build a strong team of experts in data analytics
5. Identify business uses cases, such as data fabrics, data knowledge graphs, data marketplaces, and master data management platforms.
Four Data Centric Use Cases
1. Data fabrics
More advanced organizations build data fabrics, a hyper-converged architecture focusing on integrating data across the organization’s infrastructures. Also, depending on the organization being data-centric, data Fabrics provide streamlined and secure access to disparate data across cloud and on-prem deployments, even in highly-complex distributed network environments.
2. Data knowledge graphs
Organizations create data knowledge graphs to link and leverage vast amounts of data under a common format. Data knowledge graphs need architectures to be data-centric to deliver maximum data visibility, analytics, and reuse.
3. Data marketplaces
With data marketplaces, the organizations’ golden record information can be subscribed to within a data-as-a-service framework.
4. Master data management platforms
Data centricity is an essential component of a master data management platform with a 360-degree-level view of data assets. A simple master data set or golden record can be as simple as putting data into a repository and implementing more centralized data access rules. Next-generation master data management takes the golden record and operationalizes it, having master data repositories directly power applications and analysis from the same source of truth.
Benefits of Data Centricity
Accuracy helps organizations bring efficiency to processes and helps avoid serious negative incidents
- Confidence comes from knowing that everyone is working from a common database and that the results will be based on the highest-quality data, avoiding data misunderstandings and data silos
- Consistency in the data and model across the organization ensures that different data consumers will use unified terminologies to access data, and all users will receive the same information
- Elimination of information silos makes data easily accessible and convenient for users to apply data to dynamic needs
- comes from ready access to quality data yields useful insights into internal and external trends that can affect decision making
- On-demand access allows users to tap into a centralized database when needed to perform queries and get relevant data can be accessed in whatever time zone and for whatever purpose.
- Security protocols ensure that only authorized users have access to data and provide management tools to control access
- Self-service ensures that all members of an organization can access the information they need for the precise query they are interested in answering
- Speed boosts productivity by making data available to all stakeholders when they need it, reducing the latency between questions and answers that are at the crux of keeping a project moving forward
- Unified governance of data and processes ensure the quality and depth of available data
A few additional benefits of data centricity are:
- Eliminates complex data transformations
- Facilitates more effective security and compliance
- Improves data quality and reliability
- Makes data more accessible
- Reduces data redundancy
- Reduces expense—capital and operational
- Simplifies application development, upgrades, and management
- Streamlines data management
What Is a Data Centric Model?
A data-centric model describes how data in an organization is:
- Governed
- Integrated
- Managed
- Operationalized
- Shared
- Used
Characteristics of data centricity with regards to machine learning are:
- Algorithms are fixed
- Data consistency is key
- Iteration improves data quality
- Rather than gathering more data, investments are put into data quality tools
- Working on data is the central objective
Data Centric vs Data-Driven
There is often confusion and misuse of the terms a data-centric and a data-driven approach. The direction that an organization takes is central to its culture. Not only are data-centric and data-driven not synonyms, but unchecked acquisition and analysis of data sets could easily make an organization less data-centric.
With the advent of big data and related technologies, data acquisition and processing have raised the importance of data. In the case of big data, vast amounts of information are ingested, cleaned, and analyzed using advanced analytics techniques, such as machine learning and artificial intelligence.
Organizations that have invested heavily in big data are usually data-driven with an application-centric view that focuses on a set of use cases. In these cases, the applications are responsible for collecting and storing the data they need to operate and creating data silos. This is the opposite of data centricity, where data is at the core and not the byproduct of an application that was created for a specific purpose. While big data has produced tremendous value, the data-driven approaches that are used leave out the benefits that come from taking an approach that is data-centric.
Data-Driven
A data-driven approach is a methodology for gathering, analyzing, and extracting insights from data, also referred to as analytics. Data-driven organizations use algorithms to facilitate decision-making. In terms of IT, data-driven architecture means the creation of technologies, skills, and an environment by ingesting a large amount of data. Considered a middle phase of data integration, data-driven means that the organization is using data to support decision-making processes, relying heavily on systems to make recommendations. These recommender systems rely heavily on advanced analytics, such as machine learning and artificial intelligence.
Data Centric
A data-centric approach focuses on using data as the starting point to define what should be done. Data-centric companies place data science at their core. With a data centric architecture, data is a primary and permanent asset, whereas applications change. Data-centric organizations develop and support strong cross-functional teams to build tools, develop abilities, and propagate an organization-wide culture that puts data at the heart of decision-making. To optimize the acquisition and analysis of data to make better decisions, the data-centric architecture keeps data as the primary and perpetual architectural component.
Why Is Data Centricity Important?
Data centricity is important, because it provides a common language for all the data in an organization. With everyone using the same language, communications between data users (i.e., people and applications) are more effective and efficient.
In addition, data centricity makes it possible to create optimized applications and systems maps based on the data they use and how they use it. By having these maps, data partitions are eliminated. No longer is data locked in silos aligned with the groups that generated the data.
The convergent governance of organizations’ data also enhances the quality of the information. Combined with analysis, the data can support better outcomes and decisions. It also frees users to be more creative in their thinking about how data can be used. Organizations with a data-centric culture are able to make better operational or strategic decisions. This is because the data is of the highest quality and allows users to connect the dots between information from across an organization, revealing insights and eliminating bias.
Being Data Centric Delivers Value Across the Data Value Chain
The transition from a data-driven to a data-centric business model is a significant move that requires not just technical adjustments, but a change to an organization’s mindset. To be successful, leadership and management must support the transition. This includes rallying the organization behind the adjustments that will be required across the organization’s personnel. It also requires investment in and support of the digital tools and human resources needed to support the centralization and operationalization of data.
Straightforward, timely access to information increases the value of data by enabling it to be leveraged usefully. Embracing data centricity makes decisions more reliable and provides a secure foundation for evaluating high-quality versus low-quality ideas. Going through the effort to realize Data-centricity delivers results, significantly simplifying security, integration, portability, and analysis while delivering faster insights across the entire data value chain.
Egnyte has experts ready to answer your questions. For more than a decade, Egnyte has helped more than 16,000 customers with millions of customers worldwide.
Last Updated: 2nd October, 2023