Machine Learning in the Data-driven Enterprise

Machine learning is a branch of artificial intelligence that refers to technologies that enable computers to learn and adapt through experience. It emulates human cognition - i.e. learning based on experience and patterns, rather than by inference (cause and effect). Today, deep learning advancements in machine learning allow machines to teach themselves how to build models for pattern recognition (rather than relying on humans to build them). Machine learning in the EnterpriseThe last five years have really seen a rise in AI and ML technologies for enterprises. Most of which can be attributed to advancements in computing power, and the evolution of paradigms like distributed computing, big data and cloud computing.Early commercial applications of ML were pioneered by technology titans like Google (in its search engine), Amazon (with its product recommendations) and Facebook (with its news feed). These businesses managed to build a veritable treasure trove of valuable behavioral data from hundreds of millions of users. In order to effectively collect, cleanse, organize and analyze their consumer data, these companies built scalable big data frameworks and applications then open sourced them to the world. By opening access to these big data frameworks, they improved fast, scaled quickly, and allowed businesses to derive more value from their data.From Consumer to BusinessAs big data and machine learning platforms matured and became accessible, SaaS and PaaS vendors began offering these services on the cloud at scale. The scale-on-demand paradigm and pay-as-you-go model made ML easily accessible to large and small businesses alike, including B2B business that don’t have the scale of large consumer businesses. Today, a B2B business can train data on any machine learning model, which are readily available as libraries, and with very little or no code.Key Challenges B2B companies face with Applying ML ML models are only as good as the quality and quantity of data they are trained on. Because B2B businesses don’t deal with the volumes of data that B2C businesses deal with at scale, training ML models to their requirements can be a challenge.Data privacy is another key issue for B2B businesses. While privacy is as sensitive an issue for both B2C and B2B, the liability and business impact of data misuse or leaks can be especially debilitating for B2B players. Therefore, ensuring effective access controls and anonymization of sensitive data is a critical requirement. Applying generic ML models to specific enterprise challenges may often yield questionable results. For instance, an image recognition model may be able to easily recognize generic images (e.g. buildings, automobiles etc.), but may be unable to recognize images that are unique to an industry or business (e.g. proprietary products).

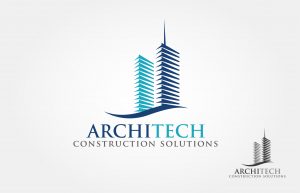
Another challenge when applying generic ML models to business problems is being able to detect anomalous trends or behaviors. For instance, a company offering a User Behavior Analytics (UBA) or enterprise collaboration solution may use ML to identify a hacked account based on an unusual access location. A generic ML model may detect a hacked account where in reality, the employee may just be traveling and working remotely. The solution therefore will need to be trained on the specific behaviors of employees in the organization.Light at the End of the TunnelDespite the significance of these challenges, there’s no reason for B2B vendors to shy away. ML (esp. DL) models get better with increased exposure to data so B2B enterprises in it for the long-term value will find ways to overcome the growing pains until their models mature. But there’s much value to be realized along the rocky road. Those that remain focused and stay the course with their ML initiatives stand to achieve a significant competitive advantage over time.Stay tuned for more articles on machine learning, covering topics like ML use cases in industries like financial services, healthcare, and construction. Also look out for our upcoming blog posts on the applications of ML in enterprise data governance, enterprise file sharing and content collaboration.
Get started with Egnyte today
Explore our unified solution for file sharing, collaboration and data governance.
LATEST PRODUCT ARTICLES
Don’t miss an update
Subscribe today to our newsletter to get all the updates right in your inbox.
By submitting this form, you are acknowledging that you have read and understand Egnyte's Privacy Policy